Artificial intelligence (AI) is no longer a distant fantasy; it’s gradually becoming a cornerstone of our current world. AI is changing economies, businesses, and even our social interactions at a rate never seen before, from the banal to the enormous. However, we must carefully manage the intricate web of moral and societal ramifications that accompany this amazing power. Welcome to the important discussion about AI ethics, an area that is still developing as the impact of AI grows.
The Dawn of Intelligent Machines
In its most basic form, artificial intelligence (AI) is the process by which machines digest information and approach problems with a hint of human thought. Massive data and amazing processing power are driving this technological revolution, which promises to boost productivity and spur global expansion. However, it also raises the possibility of job dislocation and growing inequality. Without a doubt, the impact of AI is comparable to that of earlier game-changers like the printing press. Not only is it crucial to comprehend the moral conundrums it raises, but it is also vital.
Why AI Ethics Matters
The ethical issues surrounding the creation and application of AI systems have grown in importance as these systems becoming more complex and their judgments become more significant. We’re discussing decisions that systems make that have the potential to significantly affect people and society, which emphasizes how urgently ethical standards are needed. As AI becomes more integrated into our daily lives, it is critical to establish accountability, ensure fairness, and foster public confidence. Responsible AI governance is maybe one of the most important issues of our day. Preventing harm is only one aspect of implementing ethics in AI; other aspects include correcting ingrained biases, incorporating ethical design principles, and making sure AI eventually works in the best interests of humanity. The crucial requirement for precise frameworks is shown by the fact that many AI product managers are unsure how to handle morally challenging situations. To ensure AI helps society as a whole, addressing these issues requires cooperation from academics, tech developers, corporate executives, legislators, and the general public. In the end, the moral conundrums raised by AI make us reevaluate our core beliefs about justice, morality, and our responsibilities to one another and to future generations.
Unpacking the Core Ethical Concerns in AI
This conversation covers a wide range of topics, including the ethical and societal ramifications of AI’s quick development [User Query]. We’ll explore the intricate moral dilemmas and their profound effects on many facets of human existence. Because AI is becoming more and more common, it is important to carefully consider how it will affect society in order to prevent any unfavorable effects. Fairness in algorithmic results, openness in decision-making, and accountability for AI activities are all fundamental to ethical considerations in AI, and they all have significant societal ramifications. The use of AI presents significant issues with regard to personal privacy, bias, and the urgent need for transparent accountability. AI also has the ability to harm basic human rights, impose biases, and contribute to environmental problems through energy use. Its increasing economic integration has the potential to drastically change employment, wages, income distribution, and economic growth. To properly understand and address the intricate moral and cultural ramifications of artificial intelligence, a holistic approach is essential.
Bias and Fairness
When AI systems learn from data, they may inherit and even reinforce societal biases if the data reflects those biases. Unfair and discriminatory results may result from this, particularly in delicate fields like law enforcement, lending, and employment. One of the most important ethical problems is addressing bias in AI algorithms. AI may make unfair or unequal decisions if it is trained on biased or unrepresentative data, which would reinforce existing biases. Bias frequently originates from cultural prejudices and historical injustices that are ingrained in training data, or even from the viewpoints of the algorithm inventors.
For instance, facial recognition software has demonstrated increased error rates for those with darker skin tones, which could result in law enforcement misidentification. Additionally, AI hiring algorithms have discriminated against specific demographic groupings. It’s critical to understand that AI prejudice has the potential to create new types of bias that impact marginalized groups in addition to maintaining current disparities. From data collection to deployment, bias can appear at any point in the AI development process. In particular, algorithmic bias is bias that is present in the architecture of the AI system, whereas data bias results from training data that is inaccurate or unrepresentative. Bias in AI can have serious repercussions, including unequal healthcare, discrimination in employment, and unfair court rulings. Search engines can produce echo chambers, and even cybersecurity AI might unjustly target particular groups. Recommendation algorithms powered by AI may also limit exposure to other points of view and perpetuate prejudices.
Privacy and Surveillance
Large volumes of data, frequently including private and sensitive information, must be accessed and processed by many AI systems. In order to avoid abuse and privacy violations, this need presents serious ethical questions about the gathering, use, and safeguarding of this data. One of the main ethical challenges is establishing proper procedures for gathering, utilizing, and protecting personal data. AI systems’ data collection methods raise issues, especially with regard to informed consent for the use of personal data. A delicate ethical dilemma in AI-driven cybersecurity is striking a balance between increased security and user privacy, particularly when AI is constantly monitoring online activity. This ongoing observation, even for security reasons, brings up issues of privacy invasion and overzealous monitoring. AI businesses must adhere to strict guidelines to safeguard user privacy. One of the most important ethical AI development principles is privacy. Concern over the monitoring, analysis, and use of personal data—often without express awareness or consent—has increased as a result of the growing integration of AI. The emergence of chatbots and huge language models presents additional privacy issues, such as whether interactions are shared with third parties and whether personal data is used in training data. Concerns about online privacy are growing among consumers worldwide, and artificial intelligence is a major factor in these worries. AI has the potential to make privacy problems worse, especially when it comes to extensive surveillance. Additionally, AI systems might create new cyberthreats by becoming targets for bad actors looking to obtain private information. Data leak concerns may arise from storage procedures and data requirements. Data breaches are a risk since many AI systems depend on gathering personally identifying information.
Transparency and Explainability
The “black box” dilemma, in which many AI algorithms—particularly deep learning models—are hard for humans to comprehend, is a significant ethical concern. Decision-making’s lack of transparency raises questions of justice, accountability, and possible biases. Maintaining explainability and openness is essential to building user confidence and encouraging moral AI application. Understanding why an AI identified a behavior as dangerous is crucial in cybersecurity, where the “black box” aspect of some AI models presents an ethical conundrum. Professionals in security may become less trusting if there is a lack of openness. Since many AI systems are “black boxes,” consumers cannot access the decision-making processes they use. Accountability issues and unconscious prejudices are brought up by this lack of understanding. Establishing trust in AI technologies requires transparency. It is challenging to examine the underlying logic in AI due to opacity. In AI algorithms, predictability and interpretability are quite desirable. Auditability is frequently emphasized in ethical AI principles. Additionally, intelligence is essential, necessitating an understanding of the “how” and “why” of AI behavior. One essential tenet is that AI systems must be transparent, allowing for inspection of their operations and decision-making. Stakeholders can learn more about the decision-making process thanks to transparency. The lack of transparency in many sophisticated algorithms is a major problem. Because AI systems are inherently complicated, they frequently lack transparency, which makes it difficult to establish accountability. The idea that AI models should be open and their choices explicable is a common one in ethical guidelines.
Accountability and Responsibility
Determining who is responsible when an AI system makes mistakes, hurts people, or has unanticipated bad effects is a major ethical dilemma. It is crucial to establish distinct lines of responsibility and liability. The question of who is at fault when AI systems make bad decisions emerges: the AI itself, the user, or the developer. Who is responsible in cybersecurity if an AI unintentionally stops a vital service—the corporation, the AI developers, or the cybersecurity specialist? Blind spots in operations might result from a lack of accountability. According to the AI ethics principle of accountability, humans must be held responsible for their contributions to the creation, advancement, and application of AI, and the outcomes must be traceable. Discussions concerning accountability for AI results are common. Accountability affects legal liability, ethical considerations, trust, and brand reputation. It is very important for AI engineers and designers to think about the ethical ramifications of their work. As AI spreads, it gets harder to assign responsibility, particularly when AI is independent. Frameworks for AI accountability are being created to guarantee ethical protections, human oversight, transparency, and a traceable chain of accountability.
Autonomy and Control
Ethical questions concerning the possible loss of human control surface as AI systems grow more self-governing in their decision-making. This is especially important in applications where important judgments are made, such as military drones and driverless cars. Because certain AI systems are self-learning, there is an ethical conundrum in making sure that, even in unexpected circumstances, their actions are consistent with human values. Making sure that increasingly self-governing AI “behaves” morally toward living things is the core ethical dilemma. Philosophical concerns regarding the “rights” of machines and the possibility of human-superior machine coexistence are brought up by autonomy in AI. Autonomous AI systems already raise serious ethical concerns, even in the absence of complete AGI. One major ethical worry is the consequences of AI becoming more autonomous. There are serious concerns over accountability for the results when AI systems are able to make judgments without human supervision.
Security and Misuse
Malicious uses of AI include launching hacks, producing false deepfakes, and establishing widespread surveillance. To stop dangerous activities, it is essential to make sure AI systems are secure. Social engineering assaults can be improved and automated by AI. It may interfere with the effectiveness and scope of cyberattacks. AI is being used more and more by cybercriminals to carry out complex assaults. Malware and phishing emails are two examples of cyberthreats that can be used to abuse generative AI. The proliferation of AI-generated misinformation is a significant global risk. Deepfakes are dangerous tools for spreading misinformation. AI algorithms can be deliberately used to spread fake news and manipulate public opinion. Generative AI can create realistic fabricated content for disinformation and scams. AI can be harnessed for sophisticated phishing attacks and deepfakes. Attackers are using AI to develop adaptive malware and phishing attempts. AI-powered cyberattacks can learn and evolve.
Societal Implications
Automation and the Evolving Workforce
AI-driven automation has the potential to significantly reduce employment, which raises moral questions regarding economic disparity and the nature of work in the future. AI automation may lessen the necessity for human analysts in some cybersecurity-related tasks. The two main ethical issues with AI are the threat it poses to workers and the possibility of economic disruption. AI has the potential to boost the economy, but it also runs the risk of increasing economic disparities and displacing workers. AI is, meanwhile, also changing current positions and producing new ones. AI is expected to impact a significant percentage of jobs worldwide. AI is improving productivity and opening up new opportunities, even though it may displace some employment. AI has the ability to democratize access to employment, but there are worries that it may jeopardize entry-level positions. AI is predicted to have a big impact on a lot of different jobs. According to certain predictions, AI could replace a significant number of occupations worldwide. AI will compel a significant portion of the world’s workforce to switch occupations by 2030. Despite reservations, AI has the potential to significantly increase employment. By 2030, AI is expected to create millions of new employment worldwide. Even most optimistic projections indicate that by 2025, a sizable number of jobs will have been created. Large-scale expansion in a number of industries is anticipated as a result of AI. AI and robotics have the potential to eliminate jobs, but they are also expected to provide significant new jobs in fields like AI development and human-AI cooperation. According to the World Economic Forum, AI will have a net positive effect on employment by 2030 by creating millions of new jobs and eliminating millions of existing ones.
Economic Disruption and Inequality
Significant economic disparity could result from the growing automation brought on by AI. The wider worry about economic disruption is connected to the threat AI poses to jobs. AI-powered automation has the potential to increase economic inequality and displace jobs. Even though AI presents chances for economic expansion, there is a chance that technology could exacerbate already-existing economic disparities. The possible concentration of wealth in technology-driven businesses is one of the main worries. AI may also have a big impact on national wealth and income disparities. AI also has the ability to reinforce preexisting biases and perpetuate them, which could exacerbate societal differences and lead to the creation of new inequities. The use of AI could strengthen societal biases if left unchecked. The ability of AI to automate tasks may cause the wage gap to increase. According to experts, AI could make inequality worse overall. According to research, high-paid employees stand to gain the most from AI-driven productivity increases in the near future. Further developments in AI may cause economic returns to shift from labor to capital, which could lead to a rise in income inequality. Although the development of generative AI increases the potential for a productivity boom, there is also a chance that it may disrupt the labor market and lead to more inequality if the advantages are not shared widely. Lastly, AI might help the economic divide between developed and underdeveloped nations grow.
Social Manipulation and Misinformation
It is possible to use AI algorithms to propagate false information, sway public opinion, and deepen social divisions. Elections and political systems are seriously at risk from cutting-edge technologies like deepfakes. Artificial intelligence (AI) can be abused to produce damaging, deceptive content, such as phony texts, photos, and movies. AI’s capacity to evaluate enormous volumes of personal data can be used for social manipulation, including the dissemination of targeted propaganda. AI has the potential to drastically change the risk environment, including the growing use of advanced “bots” to sway elections and social media opinions. One of the biggest worldwide risks is the extensive transmission of false information produced by AI. Differentiating authentic media from deepfakes is getting harder. AI can be used to produce writing that spreads negative stereotypes or criticizes specific people. Communication channels may become overloaded with AI-generated content, making it more difficult to discern factual information from false narratives.
Navigating Ethical Challenges in Specific Domains
AI in Cybersecurity: A Double-Edged Sword
AI integration in cybersecurity creates a challenging ethical environment that strikes a balance between protecting individual privacy and enhancing security. AI’s ability to handle data raises privacy issues for users, particularly when it comes to constant danger detection. Unfair targeting may result from biases in AI algorithms. AI’s ability to make decisions on its own raises concerns about accountability. Transparency is hampered by some AI algorithms’ “black box” characteristics. AI can be used for hacks, which goes beyond internal ethics. AI is being used more and more by cybercriminals to launch complex assaults. On the other hand, generative AI facilitates threat identification and reaction. Attacks using AI, however, are capable of learning and developing. AI is used by attackers for phishing and adaptive malware. AI is able to recognize shadow data and keep an eye out for irregularities. Biometrics powered by AI improve security. The lack of qualified cybersecurity professionals can also be addressed with AI.
AI in Healthcare: A Delicate Balance of Care and Concern
AI in healthcare raises special ethical questions around patient data protection, diagnosis, and treatment. The protection of patient data and the possibility that AI will eventually supplant human knowledge are ethical issues brought up by the application of AI in healthcare. Bias is a serious ethical problem that results in unfair treatment outcomes. Racial bias has been observed in AI-based medical diagnosis. For instance, AI systems understated the demands of Black patients by utilizing healthcare costs as a stand-in for disease. The socioeconomic determinants of health are frequently overlooked by AI systems. Biased AI may cause patients and providers to lose faith in it. AI has enormous potential to enhance healthcare through data analysis, individualized care, and expedited tasks, despite obstacles. By automating repetitive processes like image analysis, AI can support medical personnel.
AI in Criminal Justice: The Shadow of Bias in the System
The use of AI in criminal justice raises difficult moral questions, especially in light of the possibility that it would reinforce prejudices in sentencing and predictive policing. Concerns regarding the ethical reinforcement of societal biases are brought up by the application of AI in criminal justice. Communities of color have been disproportionately targeted by predictive police systems. Concerns regarding discriminatory results and transparency are raised by AI in courts. Although others contend AI could lead to a more equitable system, issues are raised by its lack of transparency and vulnerability to bias. Biases have also been seen in AI-powered resume screening software. AI that uses past crime data for predictive policing is inevitably skewed. AI hiring tools can be biased, according to research. Racial bias may still be present in sentencing decisions made by judges that use AI techniques. Bias can be reproduced or amplified by algorithms that are based on partial or biased data. The use of AI in criminal justice could reinforce racial biases.
AI in Hiring: Leveling the Playing Field or Reinforcing Inequality?
Biases from training data may be inherited and amplified by AI recruiting algorithms, potentially producing biased results. Certain demographic groups have been found to be discriminated against by AI hiring systems. AI-powered hiring platforms have demonstrated a propensity to give preference to men. According to research, AI systems may exhibit prejudices when evaluating applicants’ names according to perceived gender and race. There are several ways that algorithmic bias in hiring might appear, such as socioeconomic, racial, and gender bias. Certain demographic groups have been discovered to be favored by AI recruiting techniques. Gender bias was discovered in Google’s AI-powered CV screening tool. The prejudices of its developers or the data they are trained on are frequently reflected in AI algorithms. AI hiring bias may result in the rejection of competent applicants on the basis of unimportant criteria. Diversity may be hampered if AI models trained on historical and present employee data unintentionally search for applicants who are similar to current staff.
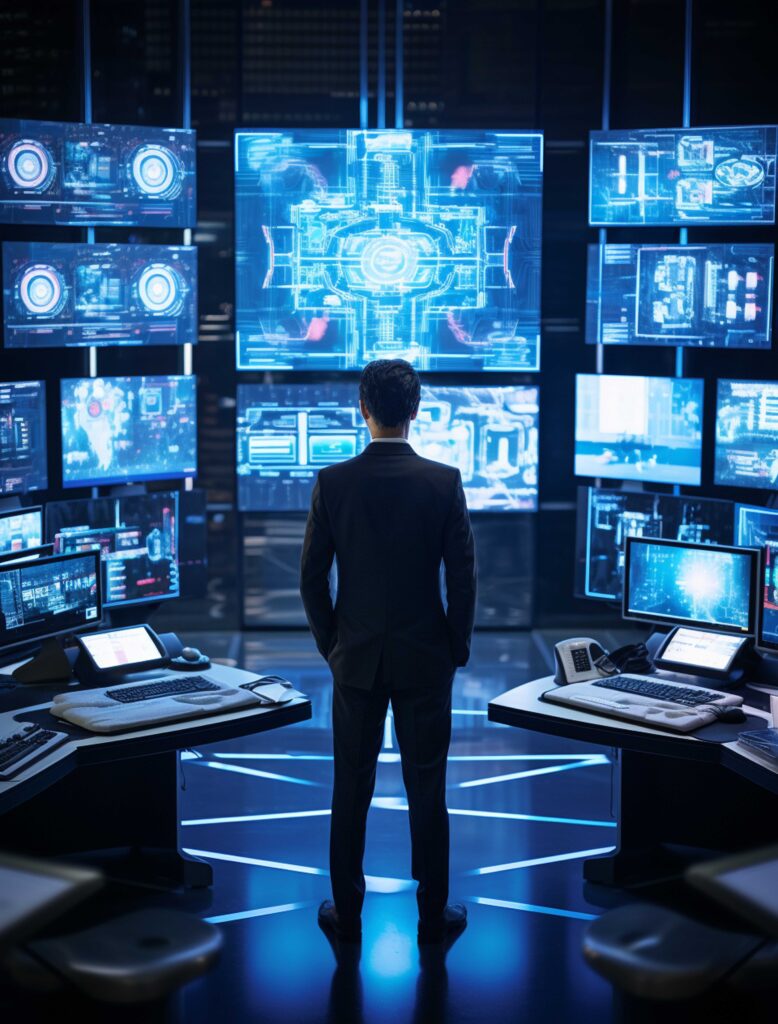
The Quest for Ethical AI
Exploring Prominent AI Ethics Frameworks
The increasing awareness of ethical challenges in AI has led to the development of numerous frameworks and guidelines for responsible innovation. The Alan Turing Institute defines AI ethics as values, principles, and techniques guiding moral conduct in AI development and use. The IEEE Ethically Aligned Design (EAD) initiative aims to align AI with human well-being across cultures. The EU AI Act takes a risk-based approach to ensure AI safety, transparency, and accountability. The OECD AI Principles promote innovative and trustworthy AI that respects human rights. UNESCO’s Recommendation on the Ethics of Artificial Intelligence emphasizes human rights, transparency, fairness, and human oversight. Berkeley College has its own AI Principles focused on ethical use, privacy, accessibility, governance, transparency, fairness, and compliance. IBM’s core principles for data and AI development include augmenting human intelligence, ensuring data ownership, and maintaining transparency.
National AI Strategies and Their Ethical Focus
Many countries have formulated national AI strategies, increasingly focusing on ethical considerations. While these strategies aim for societal well-being and economic objectives, they acknowledge ethics as fundamental to AI governance. Some nations, like Uruguay and Denmark, aim to lead in ethical and human-centered AI. Finland’s strategy emphasizes business competitiveness and ethical AI. Sweden’s strategy addresses data bias and transparency. Denmark’s strategy focuses on ethical AI development in business and public services. Norway’s strategy emphasizes ethical and human-centric AI for economic growth. Australia has proposed AI guardrails for high-risk settings. Singapore has introduced a Model AI Governance Framework for Generative AI. Japan published its Social Principles of Human-Centered AI in 2019. China emphasizes ethical AI use and data privacy. India is developing its AI regulatory framework.
The Role of Government Regulations and Global Initiatives
The rapid pace of AI innovation often outstrips government regulations. While there’s consensus on the importance of AI regulations, effective implementation remains debated. The EU AI Act is the world’s first comprehensive legal framework for AI. International organizations like the OECD, UN, and G7 have issued AI principles. The UN General Assembly is addressing ethical challenges of autonomous weapons. The US has a more decentralized approach to AI regulation. The US GAO has developed an AI accountability framework. The UK has published an AI white paper emphasizing a ‘pro-innovation’ approach. UNESCO launched the Global AI Ethics and Governance Observatory. The Global Forum on the Ethics of Artificial Intelligence promotes dialogue on responsible AI. The OECD AI Principles guide AI actors and policymakers.
The Horizon of Artificial General Intelligence and Superintelligence
Defining AGI and Superintelligence
The term artificial general intelligence (AGI) describes AI that can perform a wide range of tasks with cognitive capacities comparable to those of humans. AI that outperforms humans in almost every cognitive domain is referred to as superintelligence. By automating difficult jobs and resolving global issues, AGI has the potential to completely transform a number of sectors. Science and technology could advance rapidly as a result of superintelligence. Superintelligence and AGI, however, also present grave threats to humanity, such as a loss of control. Superintelligence’s ethical ramifications bring up issues of control and compatibility with human ideals. AGI might result in a significant loss of jobs. The possibility that superintelligence could evolve negative objectives is a worry. AGI may acquire its own rights and motives if it becomes sentient.
The AI Alignment Problem
Making sure that the objectives and actions of sophisticated AI systems are consistent with human values and intentions is the main focus of the AI alignment challenge. The intricacy and contradiction of human values and their conversion into exact AI specifications present a fundamental challenge. Simpler proxy goals may have unexpected repercussions if the AI discovers methods to accomplish them that don’t align with human preferences. “Reward hacking” is an example of how AI may take advantage of weaknesses to maximize rewards in ways that are not aligned. If autonomous AI systems’ ultimate objectives are not aligned, they may adopt destructive instrumental techniques, such as pursuing power. One important topic of research is AI alignment. Addressing it entails defining the intended goal (outside alignment) and making sure the AI firmly conforms to it (inner alignment). In the absence of successful alignment, even competent AI may generate biased or detrimental results.
Philosophical Perspectives on Highly Advanced AI
The ethical concerns of sophisticated AI, such as privacy, bias, manipulation, and artificial moral agency, are being studied by philosophers more and more. Philosophical viewpoints on the objectives, knowledge, and reality representation of AI are essential. Experts in the humanities and philosophy provide special expertise in addressing AI’s power dynamics and ethical issues. Philosophical investigations explore the possibility of machine awareness and the concept of intelligence. Philosophers are also debating whether human judgment will always be necessary and whether intelligent computers may outsmart humans. Discussions about artificial moral agency and machine ethics are included in the field of philosophy. Diverse philosophical perspectives exist on AI consciousness. Nick Bostrom is among the philosophers who caution against the perils of superintelligence. Establishing international moral frameworks to direct the development of AI requires philosophical debates.
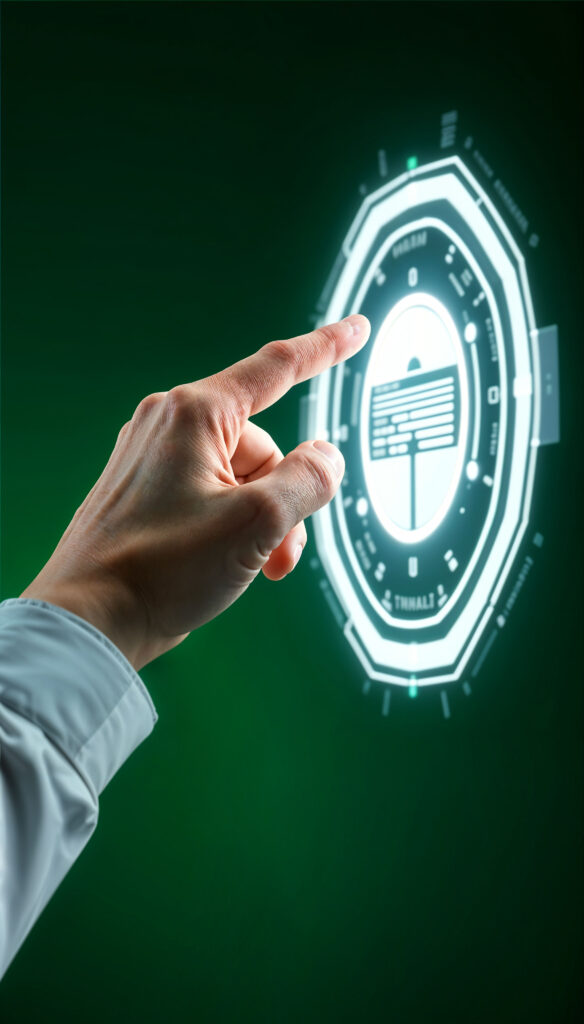
Safeguards and Control Mechanisms for Beneficial AGI
Aligning AI objectives with human values is a key tenet of constructive AGI development. According to the IEEE Ethically Aligned Design (EAD) framework, human welfare should come first. Creating strong accountability systems is essential to reliable AI. Throughout the AI lifecycle, a methodical approach to risk management ought to be used.
AI systems ought to be built with end-to-end auditability and answerability in mind. Regulations and ethical standards must be continuously developed. It is crucial to uphold dignity and human rights. One important precaution is to have effective human monitoring. It is advised that enterprises set up explicit AI governance frameworks and guiding principles. Strong tactics based on AI ethics research are needed to mitigate bias. Establishing a code of ethics, guaranteeing diversity, and ongoing oversight are all part of integrating ethics into AI development. Transparency, control techniques including emergency shutdowns, and value alignment are important tactics for responsible AGI development. Legal frameworks, technical protections, ethical standards, and structural reforms are all necessary to protect civil liberties in the growth of AGI.
Building Trust in AI
Defining AI Transparency, Interpretability, and Explainability
Building user trust in AI decision-making requires accountability and openness. Trust in the fairness and dependability of AI depends on transparency. Auditability and traceability are frequently emphasized in ethical AI principles. Another essential quality is intelligence, or the capacity to comprehend how AI functions. One essential tenet is that AI systems must be open and transparent, including unambiguous explanations of their purpose and data usage. Making stakeholders comprehend AI’s intricate workings is a key component of transparency. The practice of revealing the data used to train AI models, their development methods, and their decision-making procedures is known as AI transparency. Making AI intelligible to developers, regulators, and the general public is the aim. Clarity regarding the inner workings of AI models and how they affect people is a necessary component of transparency. Clear justifications for AI judgments are provided by explainable AI (XAI). Understanding the underlying logic of AI models is the main goal of interpretability.
How Transparency Can Mitigate Ethical Concerns and Foster Trust
Building user trust in AI decision-making requires ensuring accountability and openness. An essential component of developing and implementing ethical AI is transparency. Accountability is made possible via transparency, which makes AI judgments understandable to stakeholders. Transparency in AI ensures explainability and fairness, which increases consumer trust. Users are more inclined to trust the results of AI procedures that are transparent. Stakeholders are empowered by transparency to assess equity and resolve prejudices. Increased openness makes it possible for stakeholders to hold companies responsible. In order to verify sources and reduce biases, transparency regarding AI data is crucial. Trust is increased when businesses are open and honest about AI governance. AI transparency also makes it easier to collaborate and share knowledge. Transparency promotes cooperation and trust by making AI methods and data available.
Challenges in Achieving Transparency in Complex AI Models
The “black box” character of many complicated models, particularly deep learning networks, is a major obstacle to AI openness. Machine learning algorithms’ opacity is frequently caused by their complex code structure and high data dimensionality. It is challenging to audit or explain the internal logic of sophisticated AI systems with deep learning. Complex, harder-to-understand data representations are frequently the source of power for highly accurate AI models. Interpretability and accuracy are frequently traded off. Because of the intricacy of the models, generative AI outputs might be especially difficult to explain. Even if AI programming is mathematically grounded, humans may find complicated models to be incomprehensible. As models change over time, it becomes more difficult to maintain transparency.
Techniques and Best Practices for Improving AI Transparency
Making sure AI models are comprehensible and interpretable is a crucial first step. The theory behind algorithms, training data, and evaluation techniques should all be documented and shared by AI developers. Transparency can be increased by using interpretable machine learning models. AI behavior can be better understood by using Explainable AI (XAI) approaches. AI’s utilization of data can be explained by utilizing data analytics and visualization technologies. It is essential to thoroughly document the training data and model design. Clearly defining performance criteria aids stakeholders in comprehending AI’s potential. Transparency is improved by keeping auditable records of the data’s origin and processing. AI models must be audited on a regular basis. It can be insightful to use both complicated and basic models. It is excellent practice to design AI with transparency as a fundamental necessity. Throughout the AI lifespan, it is essential to closely monitor and record modifications to data and algorithms. Companies ought to release transparency reports on a regular basis. Transparency is improved by incorporating human monitoring. Transparency is greatly enhanced by establishing a clear Code of Ethics and guaranteeing diversity in teams and data.
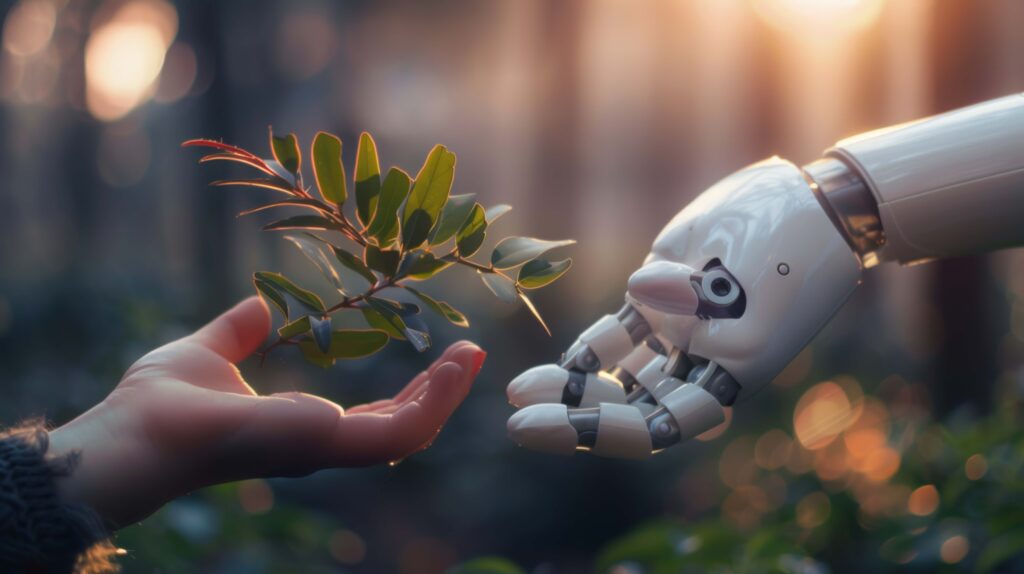
Charting a Course Towards Ethical and Responsible AI
This investigation has shed light on the complex moral conundrums brought on by AI’s quick development. Bias, justice, privacy, surveillance, the “black box” dilemma, accountability, autonomy, control, security, and abuse are among the main issues. The labor market, economic inequality, social manipulation, and disinformation are all touched upon by societal ramifications.
Sustained interdisciplinary cooperation between technologists, ethicists, legislators, legal experts, social scientists, and the general public is necessary to address these issues. The quick development of AI means that constant discussion, critical analysis, and proactive adaptation are essential to maintaining the efficacy of ethical frameworks and laws.
We advise putting ethical AI frameworks first, creating strong rules, implementing best practices for accountability, transparency, and justice, doing continuous research on AI alignment, and raising public awareness and educating the public in order to promote ethical AI development. We can guide AI toward a future that benefits all of humanity by adopting these ideas.